To make informed, data-driven decisions, one must understand the relationships and patterns in their data. Ringer Sciences specializes in discovering and interpreting these relationships for our clients, enabling them to make the best decisions for their business.
Have you ever wondered what causes stock prices to change, or how you can predict these changes? Causation and prediction, two of the hottest topics in data science, start with understanding the relationships or correlations between variables (although causation and prediction cannot be determined from these relationships alone). For instance, a first step in pursuing this inquiry would be thinking about what might be related to stock prices – like earnings, public perception, demand – and seeing whether these factors have any associations with stock prices. In fact, when a client recently approached Ringer Sciences to investigate whether certain factors were related to the stock prices of 14 energy companies, we began by computing correlations between the stock prices and potential factors of interest (chosen by our team). In addition to traditional metrics like air traffic and daily temperature, our team chose pop culture trends to explore how both related and seemingly unrelated factors might be correlated with stock prices and highlight what surprising relationships exist.
Background
Pearson’s correlation is a statistical method for describing the relationships between variables. It tells us the strength and direction of a relationship, meaning that we can see how closely variables are associated and whether they have a positive or negative relationship. Data scientists use correlations to quantify relationships because they are standardized (unlike other measures of association like covariance) and summarized in a single number, making them easy to interpret. When exploring our clients’ data, Ringer’s data science team employs correlations to identify key performance indicators (KPIs), discover connections between topics and themes, and determine new strategic avenues for campaigns.
The calculation for Pearson’s correlation coefficient includes covariance (how the two variables linearly move together around their respective means) and standard deviation (the average distance from the mean). We divide the covariance between two variables by the product of their standard deviations, which gives a standardized metric that will always range from -1 to 1.
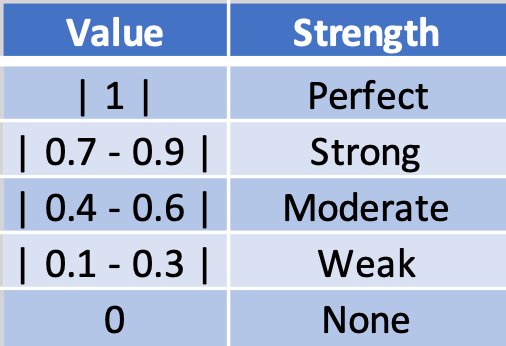
To interpret Pearson’s correlation coefficient, we always look at both the sign and value of a coefficient (denoted as r). The absolute value of r indicates strength while the sign indicates direction. The further r is from 0 (in either direction), the stronger the relationship is between the variables (Figure 1). An r close to 0 means that a pair of variables has no relationship (i.e., as one variable increases, the other variable neither increases nor decreases systematically). For instance, if the correlation between marketing spend and campaign impressions is 0.85, we would say they have a strong, positive relationship, meaning that as marketing spend increases, so do campaign impressions. An important caveat is that while correlations indicate a relationship between
variables, they don’t indicate that one variable changes because of another. This means we
can’t say for certain that marketing spending causes greater campaign impressions.
Tackling the Ask
Our client wanted to know whether certain factors were related to the stock prices of 14 different energy companies, and if so, which had the strongest relationships. The Ringer Sciences team ran Pearson correlations between the 11 different factors suggested by our team, including the daily temperature in Houston and the Google Interest Score for the term “dog food,” and the daily closing stock prices for the energy companies. Our goal was simple: to see which metric, if any, had the strongest correlation with stock prices and whether certain metrics had greater correlations with certain stocks.
First, we sourced the publicly accessible data for these 11 variables and wrangled the multiple datasets into one that we could use to calculate correlations. We retrieved most of our data from online government databases (like the National Oceanic and Atmospheric Administration and the Bureau of Transportation Statistics) and from Google. Because some of the data was only recorded monthly (like Tesla unit sales) or weekly (like Taylor Swift’s appearances on the Billboard Top 100), we calculated the average monthly, weekly, and daily stock prices for stronger comparisons. After finding the correlations, we presented our results in a heatmap table to visualize which variables had the strongest relationships with closing stock prices for the 14 companies (Figure 2.1 and Figure 2.2).
The green values show the highest positive correlations while the red values show the highest negative correlations. Each row is the daily closing stock price for a company, and each column is a factor our client was curious about.
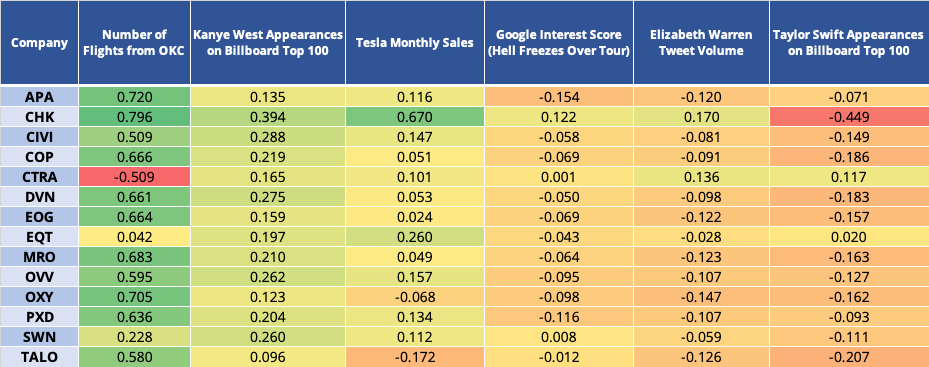
Figure 2.1: Correlation (heatmap) table. These are the 6 factors that had the highest positive correlations across the stock prices overall.
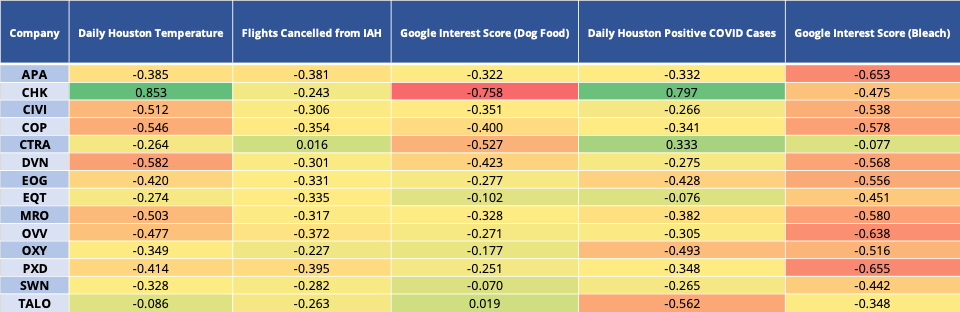
Figure 2.2: Correlation (heatmap) table. These are the 5 factors that had the highest negative correlations across the stock prices overall.
Findings
Interestingly, air traffic from the Oklahoma City airport had the strongest positive correlations overall. This means that as air traffic out of the airport increased, the stock prices across all companies rose. One possible explanation for this is that airlines needed to purchase more fuel for these higher traffic days, which boosted energy stock.
Another factor, the Google Interest Score for “bleach” (which is related to search volume), had the strongest negative correlations among most companies, indicating that as search volume for bleach increased, the stock prices for these energy companies fell. This may have been a result of the increased public interest in bleach during the COVID-19 pandemic, when transportation halted and less energy was consumed.
Not all metrics had substantial correlations. Elizabeth Warren’s tweet volume seemed to have no strong relationship with any stock prices, as the correlations for all 14 companies ranged from -0.12 to 0.15 (i.e., very close to 0). This means scrolling through Elizabeth Warren’s tweets won’t grant you much insight about the stock prices of major energy companies.
Implications
These correlations provided a better understanding of the data and new routes to explore our client’s original question. Now our client knows which factors have a relationship with which stock prices – Oklahoma City air traffic and search interest had strong-moderate relationships with the closing stock prices. Most companies’ stock prices increased as air traffic increased and as search interest in bleach decreased. This was especially true for APA, which had a strong, positive relationship with OKC air traffic and a moderately strong, negative relationship with search interest in bleach. These high correlations might compel our client to ask what causes an increase in stock prices, and with enough data, we could make predictions about when these prices will increase. By finding correlations in the data, Ringer Sciences grounds the analysis and prepares it for more informative statistical approaches like causation and forecasting that will answer the questions that matter most to our clients.
Comments